Navigating the Iceberg: Unveiling the Potential of AI in Frozen Section Pathology
Artificial intelligence (AI) is rapidly evolving as a pivotal tool in digital pathology, with its application in frozen section histopathology promising to revolutionize surgical pathology and diagnostics. Despite the inherent challenges associated with frozen sections, such as artifacts from rapid freezing, recent systematic reviews indicate a burgeoning interest in leveraging AI to enhance diagnostic accuracy and workflow efficiency in this domain. This summary aims to distill the core findings and implications of the latest research on AI’s role in frozen section pathology, providing physicians with actionable insights and underscoring the importance of continued innovation and collaboration in this field.
Key Points:
- Prevalence and Innovation in AI for Pathology: Most AI research in pathology has traditionally focused on formalin-fixed permanent sections, with frozen sections receiving less attention due to quality concerns and artifact issues.
- Systematic Review Findings: A systematic review revealed a scarcity of literature on AI applications in frozen section histopathology, underscoring a critical gap in research despite its significant potential for surgical specialties like Mohs surgery.
- AI Performance and Generalizability: Studies indicate that AI models trained on datasets including frozen sections perform better across various tissue types, highlighting the importance of diverse training sets for enhancing model generalizability.
- Challenges in AI Research and Model Evaluation: A recurring issue in AI studies is the lack of comprehensive information necessary for replicating research and assessing models’ clinical relevance, including details on model performance, bias, and generalizability.
- Comparison with Physician Diagnosis: Many studies comparing AI models to physician diagnosis in frozen section analysis do not replicate the real-life conditions of physical slide examination, potentially skewing model performance evaluations.
- Focus on Basal Cell Carcinoma (BCC): The majority of AI research in frozen sections has focused on BCC, raising questions about the clinical translational value of AI in diagnosing well-identifiable tumors with low recurrence rates.
- Impact on Patient Outcomes and Clinical Practice: The potential of AI to improve patient outcomes through enhanced diagnostic accuracy and efficiency in frozen section analysis remains a key area of interest, though its feasibility and translational value need further exploration.
- Dataset Quality and Diversity: The effectiveness of AI models is heavily dependent on the quality and diversity of the training datasets, emphasizing the need for comprehensive and representative data in model development.
- Collaborative Efforts Between AI and Clinicians: The integration of AI with clinical expertise has shown to enhance diagnostic accuracy, supporting the notion that AI should augment rather than replace physician judgment.
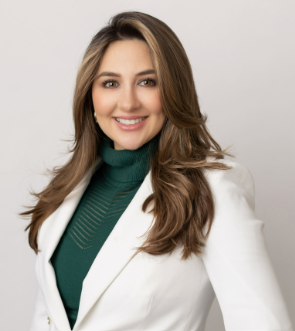
“As an optimist, I like to think that AI is going to help us all. However, I also think AI can be dangerous when not used in a patient-centric way and when physician experts are not involved in the entire process: from conception to deployment. As long as we can prioritize patients, we will be okay. But for us to do that, we need dermatologists and dermatologic surgeons to have a seat at the table.”
– Nahid Y. Vidal, MD, FAAD, FACMS, Dermatologic Surgeon, Chair of the Division of Dermatologic Surgery at Mayo Clinic in Rochester, MN
More in Dermatology