Unraveling the Unpredictable: AI Predictive Models in Clinical Settings
In the rapidly evolving landscape of AI predictive models applied to clinical settings, a simulation study reveals a cautionary tale. Insights into the potential origins of model deterioration, with implications reaching beyond ICU walls, shed light on the intricate dynamics between artificial intelligence and healthcare realities. A simulation study, centered on ICU data, exposes the vulnerability of AI predictive models. As physicians navigate the delicate balance of mortality and acute kidney injury predictions, the study unearths a disconcerting reality—AI’s attempts to refine its predictions may inadvertently undermine its own efficacy and that of fellow models.
Key Points
- Using electronic health record (EHR) data in the ICU, AI predictive models may experience “data set shift,” diminishing their performance over time.
- Changes in patient populations and practice patterns can disrupt model accuracy, as witnessed during the COVID-19 pandemic’s first wave affecting sepsis alert patterns.
- Simulation scenarios reveal that retraining models or introducing new ones can lead to significant specificity loss, ranging from 8% to 39%.
- The simultaneous implementation of acute kidney injury (AKI) and mortality prediction models may reduce each other’s effectiveness by 1% to 28%.
- Model developers are urged to simulate updating strategies at each implementation site, emphasizing the need to track predictions’ impact on clinical decisions.
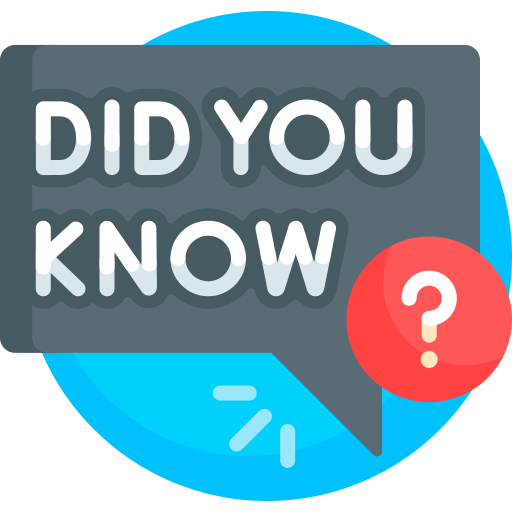
The pace of AI model evolution mirrors the challenges seen in large language models like Chat GPT. As AI becomes more intricate, monitoring and responding to model drift present critical challenges in the pursuit of precision.
More in Nephrology